Image Analysis
Image-based phenotyping has become an indispensable part of efforts to quantify the effect of genotype and environment on important plant traits. Advances in automated imaging technology now enable researchers to collect large volumes of three dimensional data, captured under visible, fluorescent and/or near-infrared light. Processing such data sets in order to extract useful information regarding a plant’s structure, its state of development or specific functional properties requires an efficient computational platform.
The focus of this research group is to develop algorithms within an integrated software environment to enable the quantitative characterisation (phenotyping) of plant morphology, development and physiology, based on multimodal and multidimensional image data derived from both high-throughput greenhouse and field experiments, and microscopic, tomographic and three dimensional scanning studies.
The tasks include structure-preserving image enhancement, supervised and unsupervised image segmentation, registration, pattern recognition and classification, computational modelling and the description of shoots, leaves, roots, spikes, seeds, tissues and cells. Staff in the group collaborate closely with plant biologists, bioinformaticians and IT scientists.
scroll top
Projects
- AVATARS - Advanced Virtuality and AugmenTed Reality AppRoaches in Seeds to Seeds (since 2019): development of algorithms for multimodal 2D/3D image registration, quantitative analysis and modeling of seed development.
- STARGATE - Sensors and daTA tRaininG towards high-performance Agri-food sysTEms (since 2021): collaboration and training in plant image analysis with EU partner organisations.
- DPPN - German Plant Phenotyping Network (2012-2019): development of algorithms and software solutions for automated shoot and root image analysis.
- Semi-automated plant image segmentation for efficient generation of ground truth data and precision phenotyping (since 2017).
- Fully automated plant image segmentation and phenotyping using pre-trained deep convolutional neural networks (since 2020).
scroll top
Staff
scroll top
Publications
Maria Antony A N, Narisetti N, Gladilin E:
Linel2D-Net: A deep learning approach to solving 2D linear elastic boundary value problems on image domains. iScience 27 (2024) 109519. https://dx.doi.org/10.1016/j.isci.2024.109519
Ullah S, Panzarová K, Trtílek M, Lexa M, Máčala V, Neumann K, Altmann T, Hejátko J, Pernisová M, Gladilin E:
High-throughput spike detection in greenhouse cultivated grain crops with attention mechanisms-based deep learning models. Plant Phenomics 6 (2024) 0155. https://dx.doi.org/10.34133/plantphenomics.0155
Xu D, Henke M, Li Y, Zhang Y, Liu A, Liu X, Li T:
Optimal design of light microclimate and planting strategy for Chinese solar greenhouses using 3D light environment simulations. Energy 302 (2024) 131805. https://doi.org/10.1016/j.energy.2024.131805
Maria Antony A N, Narisetti N, Gladilin E:
FDM data driven U-Net as a 2D Laplace PINN solver. Sci. Rep. 13 (2023) 9116. https://dx.doi.org/10.1038/s41598-023-35531-8
Narisetti N, Awais M, Khan M, Stolzenburg F, Stein N, Gladilin E:
Awn image analysis and phenotyping using BarbNet. Plant Phenomics 5 (2023) 0081. https://dx.doi.org/10.34133/plantphenomics.0081
Patil S M, Henke M, Chandramouli M, Jagarlapudi A:
Role of virtual plants in digital agriculture. In: Chaudhary S, Biradar C M, Divakaran S, Raval M S (Eds.): Digital Ecosystem for Innovation in Agriculture. (Studies in Big Data, Vol. 121) Singapore: Springer (2023) ISBN 978-981-99-0576-8, 157-182. https://dx.doi.org/10.1007/978-981-99-0577-5_8
Henke M, Gladilin E:
Virtual laser scanning approach to assessing impact of geometric inaccuracy on 3D plant traits. Remote Sens. 14 (2022) 4727. https://dx.doi.org/10.3390/rs14194727
Krause M, Neuhaus M-T, Sterker I, Bartella A K, Schönfeld A, Lethaus B, Zimmerer R, Gladilin E:
Consideration of specific key points improves outcome of decompression treatment in patients with endocrine orbitopathy: pre-/post-OP comparison and biomechanical simulation. Eur. J. Med. Res. 27 (2022) 92. https://dx.doi.org/10.1186/s40001-022-00709-9
Liu A, Henke M, Li Y, Zhang Y, Xu D, Liu X, Li T:
Investigation of the impact of supplemental reflective films to improve micro-light climate within tomato plant canopy in solar greenhouses. Front. Plant Sci. 13 (2022) 966596. https://dx.doi.org/10.3389/fpls.2022.966596
Liu A, Xu D, Henke M, Zhang Y, Li Y, Liu X, Li T:
Determination of the optimal orientation of Chinese solar greenhouses using 3D light environment simulations. Remote Sens. 14 (2022) 912. https://dx.doi.org/10.3390/rs14040912
Narisetti N, Henke M, Neumann K, Stolzenburg F, Altmann T, Gladilin E:
Deep learning based greenhouse image segmentation and shoot phenotyping (DeepShoot). Front. Plant Sci. 13 (2022) 906410. https://dx.doi.org/10.3389/fpls.2022.906410
Zhang Y, Henke M, Li Y, Xu D, Liu A, Liu X, Li T:
Analyzing the impact of greenhouse planting strategy and plant architecture on tomato plant physiology and estimated dry matter. Front. Plant Sci. 13 (2022) 828252. https://dx.doi.org/10.3389/fpls.2022.828252
Zhang Y, Henke M, Li Y, Xu D, Liu A, Liu X, Li T:
Towards the maximization of energy performance of an energy-saving Chinese solar greenhouse: A systematic analysis of common greenhouse shapes. Solar Energy 236 (2022) 320-334. https://dx.doi.org/10.1016/j.solener.2022.03.013
Blätke M-A, Beier S, Scholz U, Gladilin E, Szymanski J J (Eds.):
Front. Plant Sci., Frontiers Research Topic “Advances in Applied Bioinformatics in Crops." Lausanne: Frontiers Media SA (2021) https://dx.doi.org/10.3389/978-2-88966-620-1
Blätke M-A, Szymanski J J, Gladilin E, Scholz U, Beier S:
Editorial: Advances in applied bioinformatics in crops. Front. Plant Sci. 12 (2021) 640394. https://dx.doi.org/10.3389/fpls.2021.640394
Henke M, Neumann K, Altmann T, Gladilin E:
Semi-automated ground truth segmentation and phenotyping of plant structures using k-means clustering of eigen-colors (kmSeg). Agriculture 11 (2021) 1098. https://dx.doi.org/10.3390/agriculture11111098
Narisetti N, Henke M, Seiler C, Junker A, Ostermann J, Altmann T, Gladilin E:
Fully-automated root image analysis (faRIA). Sci. Rep. 11 (2021) 16047. https://dx.doi.org/10.1038/s41598-021-95480-y
Ullah S, Henke M, Narisetti N, Panzarová K, Trtílek M, Hejatko J, Gladilin E:
Towards automated analysis of grain spikes in greenhouse images using neural network approaches: a comparative investigation of six methods. Sensors 21 (2021) 7441. https://dx.doi.org/10.3390/s21227441
Zhang Y, Henke M, Buck-Sorlin G H, Li Y, Xu H, Liu X, Li T:
Estimating canopy leaf physiology of tomato plants grown in a solar greenhouse: Evidence from simulations of light and thermal microclimate using a Functional-Structural Plant Model. Agric. For. Meteorol. 307 (2021) 108494. https://doi.org/10.1016/j.agrformet.2021.108494
Zhen Z, Chen S, Yin T, Chavanon E, Lauret N, Guilleux J, Henke M, Qin W, Cao L, Li J, Lu P, Gastellu-Etchegorry J-P:
Using the negative soil adjustment factor of soil adjusted vegetation index (SAVI) to resist saturation effects and estimate leaf area index (LAI) in dense vegetation areas. Sensors 21 (2021) 2115. https://dx.doi.org/10.3390/s21062115
Dächert C, Gladilin E, Binder M:
Gene expression profiling of different Huh7 variants reveals novel Hepatitis C virus host Factors. Viruses 12 (2020) 36. https://doi.org/10.3390/v12010036
Gladilin E, Hierl T, Sterker I, Huempfner-Hierl H, Hemprich A, Krause M:
Retrospective cohort study of three-wall orbital resection for treatment of endocrine orbitopathy using 3D tomographic data and biomechanical modeling. Clin. Biomech. 71 (2020) 86-91. https://dx.doi.org/10.1016/j.clinbiomech.2019.10.015
Henke M, Junker A, Neumann K, Altmann T, Gladilin E:
A two-step registration-classification approach to automated segmentation of multimodal images for high-throughput greenhouse plant phenotyping. Plant Methods 16 (2020) 95. https://dx.doi.org/10.1186/s13007-020-00637-x
Kaipa J M, Starkuviene V, Erfle H, Eils R, Gladilin E:
Transcriptome profiling reveals Silibinin dose-dependent response network in non-small lung cancer cells. PeerJ 8 (2020) e10373. https://doi.org/10.7717/peerj.10373
Khosravi S, Schindele P, Gladilin E, Dunemann F, Rutten T, Puchta H, Houben A:
Application of aptamers improves CRISPR-based live imaging of plant telomeres. Front. Plant Sci. 11 (2020) 1254. https://dx.doi.org/10.3389/fpls.2020.01254
Narisetti N, Neumann K, Röder M S, Gladilin E:
Automated spike detection in diverse European wheat plants using textural features and the Frangi filter in 2D greenhouse images. Front. Plant Sci. 11 (2020) 666. https://dx.doi.org/10.3389/fpls.2020.00666
Schnurpel A:
Vergleichende Untersuchungen von Scanning-Verfahren zur Erstellung von 3D Pflanzenmodellen. (Bachelor Thesis) Mittweida, Hochschule Mittweida (2020)
Zhang Y, Henke M, Li Y, Yue X, Xu D, Liu X, Li T:
High resolution 3D simulation of light climate and thermal performance of a solar greenhouse model under tomato canopy structure. Renewable Energy 160 (2020) 730-745. https://doi.org/10.1016/j.renene.2020.06.144
Abdel-Ghani A H, Sharma R, Wabila C, Dhanagond S, Owais S J, Duwayri M A, Al-Dalain S A, Klukas C, Chen D, Lübberstedt T, von Wirén N, Graner A, Kilian B, Neumann K:
Genome-wide association mapping in a diverse spring barley collection reveals the presence of QTL hotspots and candidate genes for root and shoot architecture traits at seedling stage. BMC Plant Biol. 19 (2019) 216. https://dx.doi.org/10.1186/s12870-019-1828-5
Gladilin E, Ohse S, Boerries M, Busch H, Xu C, Schneider M, Meister M, Eils R:
TGFβ-induced cytoskeletal remodeling mediates elevation of cell stiffness and invasiveness in NSCLC. Sci. Rep. 9 (2019) 7667. https://dx.doi.org/10.1038/s41598-019-43409-x
Henke M, Junker A, Neumann K, Altmann T, Gladilin E:
Comparison and extension of three methods for automated registration of multimodal plant images. Plant Methods 15 (2019) 44. https://dx.doi.org/10.1186/s13007-019-0426-8
Henke M, Junker A, Neumann K, Altmann T, Gladilin E:
Comparison of feature point detectors for multimodal image registration in plant phenotyping. PLoS One 14 (2019) e0221203. https://dx.doi.org/10.1371/journal.pone.0221203
Hitz T, Henke M, Graeff-Honninger S, Monz S:
Three-dimensional simulation of light spectrum and intensity within an LED growth chamber. Comput. Electron. Agric. 156 (2019) 540-548. https://dx.doi.org/10.1016/j.compag.2018.11.043
Narisetti N, Henke M, Seiler C, Shi R, Junker A, Altmann T, Gladilin E:
Semi-automated Root Image Analysis (saRIA). Sci. Rep. 9 (2019) 19674. https://dx.doi.org/10.1038/s41598-019-55876-3
Chen D, Fu L Y, Hu D, Klukas C, Chen M, Kaufmann K:
The HTPmod Shiny application enables modeling and visualization of large-scale biological data. Commun. Biol. 1 (2018) 89. https://dx.doi.org/10.1038/s42003-018-0091-x
Chen D, Shi R, Pape J-M, Neumann K, Arend D, Graner A, Chen M, Klukas C:
Predicting plant biomass accumulation from image-derived parameters. GigaScience 7 (2018) giy001. https://dx.doi.org/10.1093/gigascience/giy001
Coussement J, Henke M, Lootens P, Roldán-Ruiz I, Steppe K, de Swaef T:
Modelling leaf spectral properties in a soybean functional-structural plant model by integrating the prospect radiative transfer model. Ann. Bot. 122 (2018) 669-676. https://dx.doi.org/10.1093/aob/mcy105
Guo Z, Chen D, Röder M S, Ganal M W, Schnurbusch T:
Genetic dissection of pre-anthesis sub-phase durations during the reproductive spike development of wheat. Plant J. 95 (2018) 909-918. https://dx.doi.org/10.1111/tpj.13998
Guo Z, Chen D, Schnurbusch T:
Plant and floret growth at distinct developmental stages during the stem elongation phase in wheat. Front. Plant Sci. 9 (2018) 330. https://dx.doi.org/10.3389/fpls.2018.00330
Guo Z, Zhao Y, Röder M S, Reif J C, Ganal M W, Chen D, Schnurbusch T:
Manipulation and prediction of spike morphology traits for the improvement of grain yield in wheat. Sci. Rep. 8 (2018) 14435. https://dx.doi.org/10.1038/s41598-018-31977-3
Henke M, Junker A, Neumann K, Altmann T, Gladilin E:
Automated alignment of multi-modal plant images using integrative phase correlation approach. Front. Plant Sci. 9 (2018) 1519. https://dx.doi.org/10.3389/fpls.2018.01519
Dreissig S, Schiml S, Schindele P, Weiss O, Rutten T, Schubert V, Gladilin E, Mette M F, Puchta H, Houben A:
Live cell CRISPR-imaging in plants reveals dynamic telomere movements. (The Plant Journal - 2017 Best Paper Award: Gold Prize in the category Technical Advance Article). Plant J. 91 (2017) 565-573. https://dx.doi.org/10.1111/tpj.13601
Gladilin E:
Graph-theoretical model of global human interactome reveals enhanced long-range communicability in cancer networks. PLoS One 12 (2017) e0170953. https://dx.doi.org/10.1371/journal.pone.0170953
González-Avalos P, Mürnseer M, Deeg J, Bachmann A, Spatz J, Dooley S, Eils R, Gladilin E:
Quantification of substrate and cellular strains in stretchable 3D cell cultures: an experimental and computational framework. J. Microsc. 266 (2017) 115-125. https://dx.doi.org/10.1111/jmi.12520
Guo Z, Chen D, Alqudah A M, Röder M S, Ganal M W, Schnurbusch T:
Genome-wide association analyses of 54 traits identified multiple loci for the determination of floret fertility in wheat. New Phytol. 214 (2017) 257-270. https://dx.doi.org/10.1111/nph.14342
Henke M, Buck-Sorlin G H:
Using a full spectral raytracer for the modelling of light microclimate in a functional-structural plant model. Comput. Inform. 36 (2017) 1492-1522. https://dx.doi.org/10.4149/cai_2017_6_1492
Messica Y, Laser-Azogui A, Volberg T, Elisha Y, Lysakovskaia K, Eils R, Gladilin E, Geiger B, Beck R:
The role of vimentin in regulating cell invasive migration in dense cultures of breast carcinoma cells. Nano Lett. 17 (2017) 6941-6948. https://dx.doi.org/10.1021/acs.nanolett.7b03358
Muraya M M, Chu J, Zhao Y, Junker A, Klukas C, Reif J C, Altmann T:
Genetic variation of growth dynamics in maize (Zea mays L.) revealed through automated non-invasive phenotyping. Plant J. 89 (2017) 366–380. https://dx.doi.org/10.1111/tpj.13390
Arend D, Lange M, Pape J-M, Weigelt-Fischer K, Arana-Ceballos F, Mücke I, Klukas C, Altmann T, Scholz U, Junker A:
Quantitative monitoring of Arabidopsis thaliana growth and development using high-throughput plant phenotyping. Sci. Data 3 (2016) 160055. https://dx.doi.org/10.1038/sdata.2016.55
Ćwiek-Kupczyńska H, Altmann T, Arend D, Arnaud E, Chen D, Cornut G, Fiorani F, Frohmberg W, Junker A, Klukas C, Lange M, Mazurek C, Nafissi A, Neveu P, van Oeveren J, Pommier C, Poorter H, Rocca-Serra P, Sansone S-A, Scholz U, van Schriek M, Seren Ü, Usadel B, Weise S, Kersey P, Krajewski P:
Measures for interoperability of phenotypic data: minimum information requirements and formatting. Plant Methods 12 (2016) 44. https://dx.doi.org/10.1186/s13007-016-0144-4
Pape J-M:
Ein Klassifikationssystem zur quantitativen Analyse von Krankheitssymptomen im Kontext der Hochdurchsatz-Phänotypisierung von Pflanzen. (Master Thesis) Magdeburg, Fakultät für Informatik, Otto-von-Guericke-Universität (2016) 84 pp.
Scharr H, Minervini M, French A P, Klukas C, Kramer D M, Liu X, Luengo I, Pape J-M, Polder G, Vukadinovic D, Yin X, Tsaftaris S A:
Leaf segmentation in plant phenotyping: a collation study. Mach. Vision Appl. 27 (2016) 585-606. https://dx.doi.org/10.1007/s00138-015-0737-3
Castellini A, Edlich-Muth C, Muraya M, Klukas C, Altmann T, Selbig J:
Towards a graph-theoretic approach to hybrid performance prediction from large-scale phenotypic data. In: Lones M, Tyrrell A, Smith S, Fogel G (Eds.): Information Processing in Cells and Tissues.10th International Conference, IPCAT 2015, San Diego, CA, USA, September 14-16, 2015, Proceedings. (Series: Lecture Notes in Computer Science, Vol. 9303) Cham: Springer (2015) ISBN 978-3-319-23107-5, 173-184. https://dx.doi.org/10.1007/978-3-319-23108-2_15
Guo Z, Chen D, Schnurbusch T:
Variance components, heritability and correlation analysis of anther and ovary size during the floral development of bread wheat. J. Exp. Bot. 66 (2015) 3099-3111. https://dx.doi.org/10.1093/jxb/erv117
Junker A, Muraya M M, Weigelt-Fischer K, Arana-Ceballos F, Klukas C, Melchinger A E, Meyer R C, Riewe D, Altmann T:
Optimizing experimental procedures for quantitative evaluation of crop plant performance in high throughput phenotyping systems. Front. Plant Sci. 5 (2015) 770. https://dx.doi.org/10.3389/fpls.2014.00770
Krajewski P, Chen D, Ćwiek H, van Dijk A D J, Fiorani F, Kersey P, Klukas C, Lange M, Markiewicz A, Nap J P, van Oeveren J, Pommier C, Scholz U, van Schriek M, Usadel B, Weise S:
Towards recommendations for metadata and data handling in plant phenotyping. J. Exp. Bot. 66 (2015) 5417-5427. https://dx.doi.org/10.1093/jxb/erv271
Muscolo A, Junker A, Klukas C, Weigelt-Fischer K, Riewe D, Altmann T:
Phenotypic and metabolic responses to drought and salinity of four contrasting lentil accessions. J. Exp. Bot. 66 (2015) 5467-5480. https://dx.doi.org/10.1093/jxb/erv208
Neumann K, Klukas C, Friedel S, Rischbeck P, Chen D, Entzian A, Stein N, Graner A, Kilian B:
Dissecting spatio-temporal biomass accumulation in barley under different water regimes using high-throughput image analysis. Plant Cell Environ. 38 (2015) 1980-1996. https://dx.doi.org/10.1111/pce.12516
Pape J M, Klukas C:
Utilizing machine learning approaches to improve the prediction of leaf counts and individual leaf segmentation of rosette plant images. In: Tsaftaris S A, Scharr H, Pridmore T (Eds.): Proceedings of the Computer Vision Problems in Plant Phenotyping (CVPPP). : BMVA Press (2015) ISBN 1-901725-55-3, 3.1-3.12. https://dx.doi.org/10.5244/C.29.CVPPP.3
Pape J M, Klukas C:
3-D histogram-based segmentation and leaf detection for rosette plants. In: Fleet D, Pajdla T, Schiele B, Tuytelaars T (Eds.): Computer Vision – ECCV 2014 Workshops: 13th European Conference, Zurich, Switzerland, September 6-12, 2014, proceedings, part IV (Series: Lecture Notes in Computer Science) Cham: Springer (2015) ISBN 978-3-319-16219-5, 61-74. https://doi.org/10.1007/978-3-319-16220-1_5
Rahaman M M, Chen D, Gillani Z, Klukas C, Chen M:
Advanced phenotyping and phenotype data analysis for the study of plant growth and development. Front. Plant Sci. 6 (2015) 619. https://dx.doi.org/10.3389/fpls.2015.00619
Schilling S, Gramzow L, Lobbes D, Kirbis A, Weilandt L, Hoffmeier A, Junker A, Weigelt-Fischer K, Klukas C, Wu F, Meng Z, Altmann T, Theissen G:
Non-canonical structure, function and phylogeny of the B MADS-box gene OsMADS30 of rice (Oryza sativa). Plant J. 84 (2015) 1059-1072. https://dx.doi.org/10.1111/tpj.13055
Yuan C, Wang J, Harrison A P, Meng X, Chen D, Chen M:
Genome-wide view of natural antisense transcripts in Arabidopsis thaliana. DNA Res. 22 (2015) 233-243. https://dx.doi.org/10.1093/dnares/dsv008
Chen D, Chen M, Altmann T, Klukas C:
Bridging genomics and phenomics. In: Chen M, Hofestädt R (Eds.): Approaches in integrative bioinformatics: towards the virtual cell. Berlin [u.a.]: Springer (2014) ISBN 978-3-642-41280-6, 299-333. https://dx.doi.org/10.1007/978-3-642-41281-3_11
Chen D, Fu L Y, Zhang Z, Li G, Zhang H, Jiang L, Harrison A P, Shanahan H P, Klukas C, Zhang H Y, Ruan Y, Chen L L, Chen M:
Dissecting the chromatin interactome of microRNA genes. Nucleic Acids Res. 42 (2014) 3028-3043. https://dx.doi.org/10.1093/nar/gkt1294
Chen D, Neumann K, Friedel S, Kilian B, Chen M, Altmann T, Klukas C:
Dissecting the phenotypic components of crop plant growth and drought responses based on high-throughput image analysis. Plant Cell 26 (2014) 4636-4655. https://dx.doi.org/10.1105/tpc.114.129601
Harshavardhan V T, Van Son L, Seiler C, Junker A, Weigelt-Fischer K, Klukas C, Altmann T, Sreenivasulu N, Bäumlein H, Kuhlmann M:
AtRD22 and AtUSPL1, members of the plant-specific BURP domain family involved in Arabidopsis thaliana drought tolerance. PLoS One 9 (2014) e110065. https://dx.doi.org/10.1371/journal.pone.0110065
Klukas C, Chen D, Pape J M:
Integrated Analysis Platform: an open-source information system for high-throughput plant phenotyping. Plant Physiol. 165 (2014) 506-518. https://dx.doi.org/10.1104/pp.113.233932
Chen D, Chen M, Klukas C:
Phenomics collaboration website. phenomics.cn (2013).
Klukas C, Chen D, Pape J-M:
IAP – The Integrated Analysis Platform for High-Througput Plant Phenotyping. iap.ipk-gatersleben.de (2013).
Lu X, Chen D, Shu D, Zhang Z, Wang W, Klukas C, Chen L L, Fan Y, Chen M, Zhang C:
The differential transcription network between embryo and endosperm in the early developing maize seed. Plant Physiol. 162 (2013) 440-455. https://dx.doi.org/10.1104/pp.113.214874
Rohn H, Junker A, Hartmann A, Grafahrend-Belau E, Treutler H, Klapperstück M, Czauderna T, Klukas C, Schreiber F:
Vanted 2.0. www.vanted.org (2013).
Junker A, Rohn H, Czauderna T, Klukas C, Hartmann A, Schreiber F:
Creating interactive, web-based and data-enriched maps with the Systems Biology Graphical Notation. Nat. Protoc. 7 (2012) 579-593. https://dx.doi.org/10.1038/nprot.2012.002
Jusufi I, Klukas C, Kerren A, Schreiber F:
Guiding the interactive exploration of metabolic pathway interconnections. Inform. Visual. 11 (2012) 136-150. https://dx.doi.org/10.1177/1473871611405677
Klukas C, Pape J M, Entzian A:
Analysis of high-throughput plant image data with the information system IAP. J. Integr. Bioinform. 9 (2012) 191. https://dx.doi.org/10.2390/biecoll-jib-2012-191
Neumann K, Stein N, Graner A, Klukas C, Entzian A, Kilian B:
Non-destructive phenotyping using the high-throughput LemnaTec-Scanalyzer 3D platform to investigate drought tolerance in barley. In: Börner A, Kobijlski B (Eds.): Proceedings of the 15th International EWAC Conference, 7 - 11 November 2011, Novi Sad, Serbia. (Series: European Wheat Aneuploid Co-operative newsletter, Vol. 15) Gatersleben: Leibniz-Institut für Pflanzengenetik und Kulturpflanzenforschung (2012) 158-160.
Rohn H, Junker A, Hartmann A, Grafahrend-Belau E, Treutler H, Klapperstück M, Czauderna T, Klukas C, Schreiber F:
Vanted 2.0. www.vanted.org (2012).
Rohn H, Junker A, Hartmann A, Grafahrend-Belau E, Treutler H, Klapperstück M, Czauderna T, Klukas C, Schreiber F:
VANTED v2: a framework for systems biology applications. BMC Syst. Biol. 6 (2012) 139. https://dx.doi.org/10.1186/1752-0509-6-139
Huang D, Huang Y, Bai Y, Chen D, Hofestädt R, Klukas C, Chen M:
MyBioNet: interactively visualize, edit and merge biological networks on the Web. Bioinformatics 27 (2011) 3321-3322. https://dx.doi.org/10.1093/bioinformatics/btr557
Pape J-M:
Automatische Erkennung und Quantifizierung von phänotypischen Pflanzenmerkmalen und Strukturen. (Bachelor Thesis) Magdeburg, Fakultät für Informatik, Otto-von-Guericke-Universität (2011) 67 pp.
Rohn H, Klukas C, Schreiber F:
Vanted 2.0. www.vanted.org (2011).
Rohn H, Klukas C, Schreiber F:
Creating views on integrated multidomain data. Bioinformatics 27 (2011) 1839-1845. https://dx.doi.org/10.1093/bioinformatics/btr282
Rohn H, Klukas C, Schreiber F:
Visual analytics of multimodal biological data. Proceedings of the International Conference on Information Visualization Theory and Applications (IVAPP’11). SciTePress (2011) 256-261.
Weise S, Grosse I, Klukas C, Koschützki D, Scholz U, Schreiber F, Junker B H:
Meta-All: A system for managing metabolic pathway information. meta-all.ipk-gatersleben.de (2011).
Czauderna T, Klukas C, Schreiber F:
SBGN-ED. vanted.ipk-gatersleben.de/addons/sbgn-ed/ (2010).
Czauderna T, Klukas C, Schreiber F:
Editing, validating and translating of SBGN maps. Bioinformatics 26 (2010) 2340-2341. https://dx.doi.org/10.1093/bioinformatics/btq407
Hippe K, Colmsee C, Czauderna T, Grafahrend-Belau E, Junker B H, Klukas C, Scholz U, Schreiber F, Weise S:
Novel developments of the MetaCrop information system for facilitating systems biological approaches. J. Integr. Bioinform. 7 (2010) e125. https://dx.doi.org/10.2390/biecoll-jib-2010-125
Huang D, Huang Y, Klukas C, Hofestädt R, Chen M:
PBSK Browser: navigate biological pathways of PSI-MI, BioPAX, SBML and KGML formats. In: di Bernado D (Ed.): IEEE International Conference on Bioinformatics and Biomedicine workshops (BIBMW 2010): Hong Kong, China, 18 - 21 December 2010, proceedings. Piscataway, NJ: IEEE (2010) ISBN 978-1-4244-8303-7, 13-18. https://dx.doi.org/10.1109/BIBMW.2010.5703766
Klukas C, Schreiber F:
VANTED. vanted.ipk-gatersleben.de (2010).
Klukas C, Schreiber F:
Integration of -omics data and networks for biomedical research with VANTED. J. Integr. Bioinform. 7 (2010) 112. https://dx.doi.org/10.2390/biecoll-jib-2010-112
Rohn H, Klukas C, Schreiber F:
HIVE. vanted.ipk-gatersleben.de/addons/hive/ (2010).
Sharbel T F, Voigt M L, Corral J M, Galla G, Kumlehn J, Klukas C, Schreiber F, Vogel H, Rotter B:
Apomictic and sexual ovules of Boechera display heterochronic global gene expression patterns (Hofmann, N.R.: Apomixis and gene expression in Boechera. Plant Cell (2010) 22:539. Highlight of Sharbel et al. 2010 by Plant Cell scientific editor). Plant Cell 22 (2010) 655-671. https://dx.doi.org/10.1105/tpc.109.072223
Weidemann W, Klukas C, Klein A, Simm A, Schreiber F, Horstkorte R:
Lessons from GNE-deficient embryonic stem cells: Sialic acid biosynthesis is involved in proliferation and gene expression. Glycobiology 20 (2010) 107-117. https://dx.doi.org/10.1093/glycob/cwp153
Weise S, Grosse I, Klukas C, Koschützki D, Scholz U, Schreiber F, Junker B H:
Meta-All: A system for managing metabolic pathway information. meta-all.ipk-gatersleben.de (2010).
Grafahrend-Belau E, Junker B H, Klukas C, Koschützki D, Schreiber F, Schwöbbemeyer H:
Topology of plant metabolic networks. In: Schwender J (Ed.): Plant metabolic networks. Dordrecht [u.a.]: Springer (2009) ISBN 978-0-387-78744-2, 173-209. https://dx.doi.org/10.1007/978-0-387-78745-9_7
Grafahrend-Belau E, Klukas C, Junker B H, Schreiber F:
FBASimViz: dynamic visualization of constraint-based metabolic models. fbasimviz.ipkgatersleben.de (2009).
Grafahrend-Belau E, Klukas C, Junker B H, Schreiber F:
FBA-SimVis: Interactive visualisation of constraint-based metabolic models. Bioinformatics 25 (2009) 2755-2757. https://dx.doi.org/10.1093/bioinformatics/btp408
Klukas C:
Analyse und Visualisierung von Experimentdaten im Kontext biologischer Netzwerke. (PhD Thesis) Halle/S., Martin-Luther-Universität Halle-Wittenberg (2009) 100 pp.
Klukas C, Rohn H, Schreiber F:
Vanted and Vanted3D. vanted.ipk-gatersleben.de (2009).
Klukas C, Schreiber F:
Integration of -omics data and networks for biomedical research. In: Proceedings of the International Workshop in Computational & Integrative Biology: a satellite meeting of the International Conference of Integrative Biology, 18.-20.09.2009, Hangzhou, China. Hanzhou (2009) 14-19.
Rohn H, Klukas C, Schreiber F:
Integration and visualisation of multimodal biological data. In: German Conference on Bioinformatics 2009: 28 - 30 September 2009, Martin Luther University Halle-Wittenberg, Germany, proceedings (Series: Lecture notes in informatics, Vol. 157) Bonn: Ges. für Informatik (2009) ISBN 978-3-88579-251-2, 105-115.
Weise S, Colmsee C, Grafahrend-Belau E, Junker B, Klukas C, Lange M, Scholz U, Schreiber F:
Datenaustausch und Datenintegration zur Modellierung und Analyse metabolischer Netzwerke am Beispiel von Kulturpflanzen. In: Fischer S, Maehle E, Reischuk R (Eds.): Informatik 2009: im Focus das Leben, Beiträge der 39. Jahrestagung der Gesellschaft für Informatik e.V. (GI), 28.9. - 2.10.2009 in Lübeck. (Series: GI-Edition, Lecture notes in informatics, Vol. 154) Bonn: Ges. für Informatik (2009) ISBN 978-3-88579-248-2, 693-697.
Weise S, Colmsee C, Grafahrend-Belau E, Junker B, Klukas C, Lange M, Scholz U, Schreiber F:
An integration and analysis pipeline for systems biology in crop plant metabolism. In: Paton N W, Missier P, Hedeler C (Eds.): Data integration in the life sciences: 6th international workshop, DILS 2009, Manchester, UK, July 20-22, 2009, proceedings. (Series: Lecture Notes in Computer Science, Vol. 5647) Berlin [u.a.]: Springer (2009) ISBN 978-3-642-02878-6, 196-203. https://dx.doi.org/10.1007/978-3-642-02879-3_16
Wheelock C E, Goto S, Yetukuri L, DAlexandri F L, Klukas C, Schreiber F, Oresic M:
Bioinformatics strategies for the analysis of lipids. In: Armstrong D (Ed.): Lipidomics, Vol. 2: methods and protocols. (Series: Methods in molecular biology, Vol. 580) New York, NY: Humana Press (2009) ISBN 978-1-60761-324-4, 339-368. https://dx.doi.org/10.1007/978-1-60761-325-1_19
Grafahrend-Belau E, Junker B H, Koschützki D, Klukas C, Weise S, Scholz U, Schreiber F:
Towards systems biology of developing barley grains: a framework for modeling metabolism. In: Ahdesmäki M, Strimmer K, Radde N, Rahnenführer J, Klemm K, Lähdesmäki H, Yli-Harja O (Eds.): 5th International Workshop on Computational Systems Biology, WCSB 2008, June 11–13, 2008, Leipzig, Germany, proceedings. (TICSP series, Vol. 41) Tampere: Tampere International Center for Signal Processing (2008) ISBN 978-952-15-1988-8 41-44.
Klukas C:
VANTED - visualization and analysis of networks containing experimental data. vanted.ipk-gatersleben.de (2008).
Weise S, Grosse I, Klukas C, Koschützki D, Scholz U, Schreiber F, Junker B H:
Meta-All: A system for managing metabolic pathway Information. meta-all.ipk-gatersleben.de (2008).
Klukas C:
VANTED - visualization and analysis of networks containing experimental data. vanted.ipk-gatersleben.de (2007).
Klukas C, Schreiber F:
Dynamic exploration and editing of KEGG pathway diagrams. Bioinformatics 23 (2007) 344-350. https://dx.doi.org/10.1093/bioinformatics/btl611
Rolletschek H, Nguyen T H, Häusler R E, Rutten T, Göbel C, Feussner I, Radchuk R, Tewes A, Claus B, Klukas C, Linemann U, Weber H, Wobus U, Borisjuk L:
Antisense inhibition of the plastidial glucose-6-phosphate/phosphate translocator in Vicia seeds shifts cellular differentiation and promotes protein storage. Plant J. 51 (2007) 468-484. https://dx.doi.org/10.1111/j.1365-313X.2007.03155.x
Junker B H, Klukas C, Schreiber F:
VANTED: a system for advanced data analysis and visualization in the context of biological networks. BMC Bioinformatics 7 (2006) 109. https://dx.doi.org/10.1186/1471-2105-7-109
Junker B H, Klukas C, Schreiber F:
VANTED: Datenauswertung im Netzwerk-Kontext. GenomXPress 1 (2006) 16-18.
Klukas C:
KGML-ED - a graphical KGML pathway editor. kgml-ed.ipk-gatersleben.de (2006).
Klukas C, Junker B H, Schreiber F:
The VANTED software system for transcriptomics, proteomics and metabolomics analysis. J. Pestic. Sci. 31 (2006) 289-292.
Klukas C, Schreiber F:
VANTED - visualization and analysis of networks containing experimental data. vanted.ipk-gatersleben.de (2006).
Klukas C, Schreiber F, Schwöbbermeyer H:
Coordinated perspectives and enhanced force-directed layout for the analysis of network motifs. In: Misue K, Sugiyama K, Tanaka J (Eds.): Information Visualisation 2006: proceedings of the Asia Pacific Symposium on Information Visualisation (APVIS2006). (Series: Conferences in research and practice in information technology, Vol. 60) Tokyo: ACS (2006) ISBN 1-920682-41-4, 39-48.
Weise S, Grosse I, Klukas C, Koschützki D, Scholz U, Schreiber F, Junker B H:
Meta-All: a system for managing metabolic pathway information. BMC Bioinformatics 7 (2006) 465. https://dx.doi.org/10.1186/1471-2105-7-465
scroll top
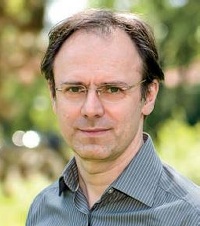